SVLearn: a dual-reference machine learning approach enables accurate cross-species genotyping of structural variants
Mar 11, 2025·
,,,,,,,,,,,,,,,,·
1 min read
Qimeng Yang

Jianfeng Sun
Xinyu Wang
Jiong Wang
Quanzhong Liu
Jinlong Ru
Xin Zhang
Sizhe Wang
Ran Hao
Peipei Bian
Xuelei Dai
Mian Gong
Zhuangbiao Zhang
Ao Wang
Fengting Bai
Ran Li
Yudong Cai
Yu Jiang
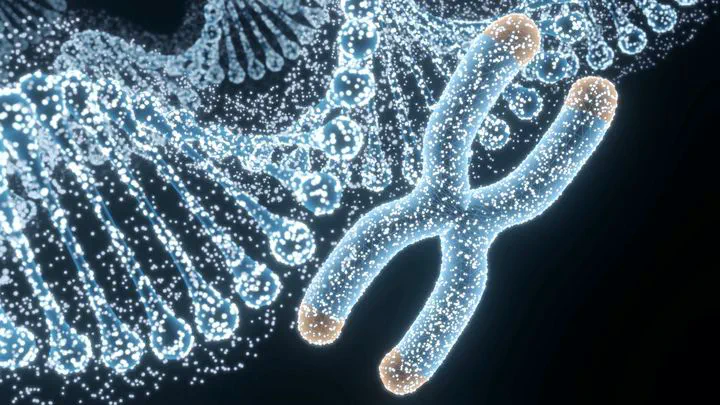
Abstract
Structural variations (SVs) are diverse forms of genetic alterations and drive a wide range of human diseases. Accurately genotyping SVs, particularly occurring at repetitive genomic regions, from short-read sequencing data remains challenging. Here, we introduce SVLearn, a machine-learning approach for genotyping bi-allelic SVs. It exploits a dual-reference strategy to engineer a curated set of genomic, alignment, and genotyping features based on a reference genome in concert with an allele-based alternative genome. Using 38,613 human-derived SVs, we show that SVLearn significantly outperforms four state-of-the-art tools, with precision improvements of up to 15.61% for insertions and 13.75% for deletions in repetitive regions. On two additional sets of 121,435 cattle SVs and 113,042 sheep SVs, SVLearn demonstrates a strong generalizability to cross-species genotype SVs with a weighted genotype concordance score of up to 90%. Notably, SVLearn enables accurate genotyping of SVs at low sequencing coverage, which is comparable to the accuracy at 30× coverage. Our studies suggest that SVLearn can accelerate the understanding of associations between the genome-scale, high-quality genotyped SVs and diseases across multiple species.
Type
Publication
Nature Communications