ICMLC 2019
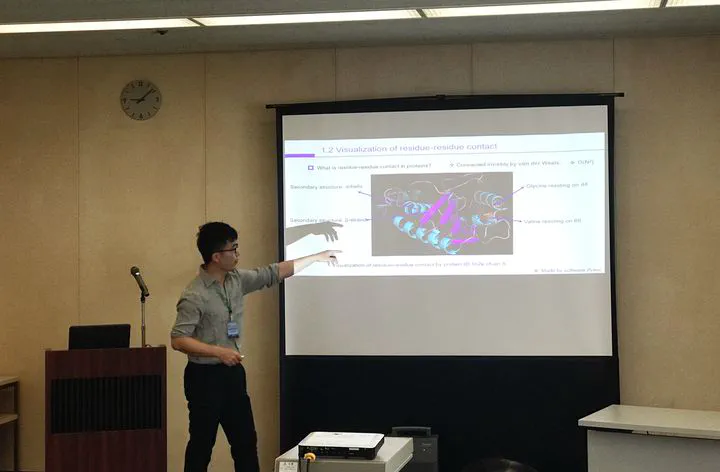
Kobe Convention Center
6 Chome, Kobe, Minatojima Nakamachi 650-0046
At this conference, I focused on only one question: classification effects of contacting and non-contacting residue pairs using dimensionality reduction techniques.
My aim was to evaluate whether the features defined for residue pairs are effective in distinguishing between these two categories. Specifically, I sought to determine if the data could be meaningfully separated in a two-dimensional space. To investigate this, I employed several computational approaches for visualizing the separability of the data.
For the autoencoder, I implemented a deep architecture with four layers each in the encoder and decoder, compressing from 256 hidden neurons down to a 2-dimensional latent space. For PCA and t-SNE, I directly projected the data into two dimensions to facilitate comparison.
Across all three unsupervised methods, I observed that contacting and non-contacting residue pairs could be broadly separated. Notably, the dispersed nature of non-contacting residue pairs became increasingly pronounced in most visualizations. In particular, the t-SNE results revealed a clearer separation, with residue pairs forming two distinct clusters.